Finding the correct information at the right time is a pressing challenge for companies, as SaaS sprawl increases and the amount of data grows. Retrieval-Augmented Generation, or RAG search, is an AI-driven approach that solves this problem by combining advanced search with AI models’ generative capabilities.
As organizations move toward more intelligent workflows and data-driven decisions, RAG search offers a powerful tool to enhance knowledge management, improve operational efficiency, and foster better decision-making.
What is RAG Search?
Retrieval-Augmented Generation (RAG) is an AI methodology that enhances traditional information retrieval by pairing it with generative AI. Unlike standalone search engines or generative AI systems alone, RAG offers the best of both worlds. It retrieves relevant information from trusted sources and generates a natural language response, grounded in the retrieved data.
This dual approach provides more accurate and context-aware answers compared to conventional systems.
Critical components of RAG search include:
- Retrieval: The AI fetches relevant documents or data points from a pre-defined knowledge base or database using vector similarity or semantic search techniques.
- Generation: A generative AI model, such as OpenAI’s ChatGPT, synthesizes a coherent, human-like response based on the retrieved information.
RAG search ensures that the AI can handle complex queries, generate nuanced answers, and ground its responses in factual data, reducing inaccuracies or hallucinations.
How Does RAG Search Work?
RAG search operates in two phases, leveraging advanced AI technologies for each step:
1. Retrieval Phase
When a user poses a query, the system:
- Transforms the query into vector embeddings, representing the text’s semantic meaning.
- Searches a database or knowledge base using similarity metrics for the most contextually relevant documents.
- Selects top-ranked documents or data points to feed into the next phase.
This retrieval process relies on semantic search rather than simple keyword matching, allowing it to understand intent and context better.
2. Generation Phase
Once the documents are retrieved, the generative AI:
- Takes these documents as input to craft a response.
- Uses contextual information from the query and the documents to generate a response in natural language.
The result is a conversational, accurate, and context-aware response tailored to the user’s needs.
Value of RAG to Enterprises
RAG search holds immense potential for organizations looking to streamline operations, enhance employee productivity, and deliver superior customer experiences.
1. Enhanced Knowledge Access
RAG enables employees to access precise information quickly, eliminating the need to search through extensive documentation. Whether retrieving compliance details, IT solutions, or HR policies, RAG ensures teams have the answers they need at their fingertips.
2. Improved Customer Support
Companies can provide personalized and context-aware support by integrating RAG into customer service platforms. Customers receive quick, accurate responses, which improves satisfaction and reduces the support team workload.
3. Increased Productivity
Automating information retrieval reduces time spent searching for data, enabling teams to focus on strategic tasks. For example, a marketing team could instantly find data on campaign performance or customer demographics without manual effort.
4. Actionable Insights
RAG search enables decision-makers to receive actionable, reliable insights by grounding generative outputs in real-time or pre-validated data. This helps in crafting data-driven strategies across departments.
5. Scalability and Adaptability
RAG’s flexible framework allows it to adapt to diverse use cases, including:
- HR: Answering employee queries about benefits or onboarding processes.
- IT: Find the knowledge and resources to resolve issues faster.
- Engineering: Surface issues, context, and collaborators with just a search.
- Marketing: Optimizing workflows to maximize impact and reach.
- Product: Surface roadmaps, tasks, release documentation, and related work.
GoSearch: Unlocking the Power of RAG for Enterprises
At the forefront of implementing RAG is GoSearch, an advanced AI-driven search solution tailored to enterprise needs. GoSearch integrates retrieval-augmented generation to offer businesses a seamless and efficient search experience. Here’s how it delivers value:
1. Unified Knowledge Repository
GoSearch connects disparate data sources, such as intranets, CRM systems, and cloud storage, into a unified knowledge base. Employees can surface accurate answers without navigating multiple platforms.
2. Context-Aware Results
Using RAG search, GoSearch delivers relevant responses that are also enriched with context. It provides synthesized answers that directly address user queries instead of simply listing documents.
3. Enhanced Decision-Making
With GoSearch, organizations can extract actionable insights from unstructured data, enabling leaders to make informed decisions.
4. Scalability Across Teams
From assisting IT teams in troubleshooting to empowering HR with instant access to company policies, GoSearch scales to fit the needs of diverse departments.
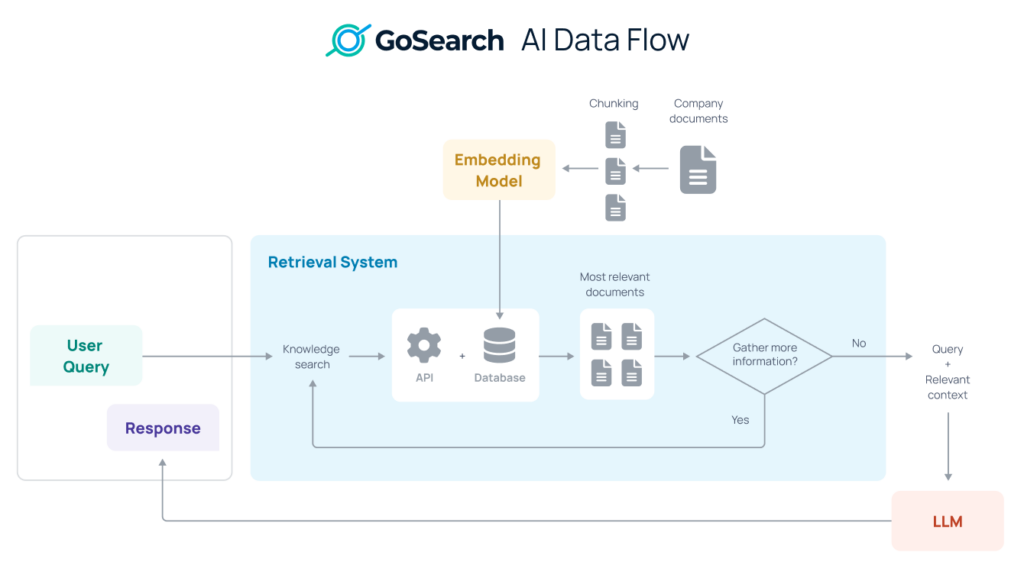
What’s Next for RAG Search?
The future of RAG is bright, with several exciting advancements on the horizon:
1. Integration with Real-Time Data
RAG systems will soon incorporate real-time data sources, allowing businesses to respond to dynamic changes. This is particularly useful for industries like finance, where up-to-the-minute information is crucial.
2. Enhanced Personalization
As AI becomes more adept at understanding user preferences, RAG will deliver highly personalized results tailored to individual needs, further improving user satisfaction.
3. Industry-Specific Applications
RAG will evolve to offer specialized solutions for industries like healthcare (medical document retrieval), education (personalized learning resources), and e-commerce (tailored product recommendations).
4. Improved AI Explainability
Future RAG models will likely include better mechanisms to explain how a response was generated and what data sources were used, increasing trust and transparency.
5. Hybrid Cloud Implementations
Enterprises will have the flexibility to deploy RAG systems in hybrid environments, ensuring data privacy and compliance with industry regulations.
Why Enterprises Should Embrace RAG Search
Retrieval-Augmented Generation, or RAG search, stands out as a game-changer in a data-driven world. By combining the accuracy of retrieval-based systems with the conversational power of generative AI, RAG offers unparalleled benefits for enterprises.
Tools like GoSearch make adopting RAG seamless and scalable, empowering organizations to enhance productivity, improve customer experiences, and drive innovation. As RAG search evolves, its potential to transform industries will only grow, making now the perfect time for enterprises to invest in RAG-powered solutions.
Whether you’re looking to streamline workflows, foster collaboration, or make better-informed decisions, RAG search is the key to unlocking a more innovative, efficient future.
Search across all your apps for instant AI answers with GoSearch
Schedule a demo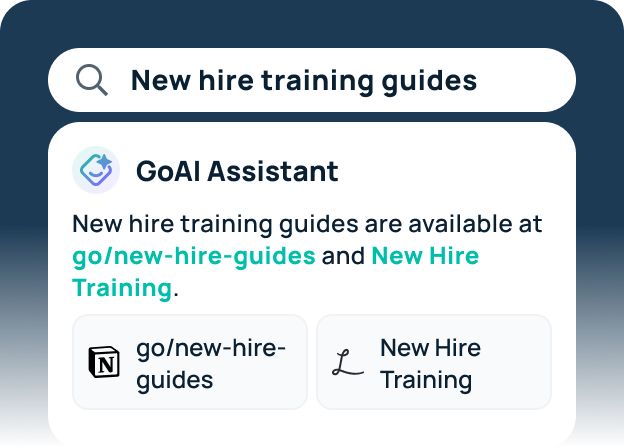
FAQs
1. What is RAG?
Retrieval-Augmented Generation (RAG) is a technique that combines information retrieval systems with generative AI models to deliver accurate, context-aware answers. It works by first retrieving relevant documents from a knowledge base and then using a generative AI model (like ChatGPT) to synthesize a natural language response grounded in the retrieved data. This ensures responses are both informative and accurate, minimizing AI hallucinations.
2. How does RAG improve traditional search systems?
RAG enhances traditional search systems by pairing keyword or semantic retrieval with AI’s generative capabilities. Instead of simply listing documents or links, RAG delivers synthesized, conversational responses that directly address user queries. This improves user experience by providing contextually relevant answers, saving time and reducing the cognitive load of processing raw data.
3. What are the main use cases of RAG in enterprises?
RAG has diverse applications, including:
- Enterprise Knowledge Management: Efficiently retrieve and synthesize insights from internal knowledge bases, wikis, or documentation.
- Customer Support & Chatbots: Power chatbots to answer complex customer queries using real-time retrieval from FAQs, ticket histories, or support databases.
- Content Generation: Automatically generate reports, proposals, or presentations enriched with relevant, real-time data.
- Data-Driven Decision Support: Provide decision-makers with contextualized, synthesized insights from structured and unstructured data sources.
- Employee Onboarding and Training: Provide new employees with personalized, on-demand access to company resources, training materials, and process guides.
4. How does RAG ensure accuracy in AI-generated responses?
RAG ensures accuracy by grounding the generative model’s output in data retrieved from verified sources. The retrieval step narrows the AI’s input to a set of high-relevance documents, minimizing the chances of hallucinations (incorrect or made-up information). Additionally, many RAG systems include mechanisms to cite sources, further boosting trust and transparency.
5. What’s the difference between RAG and traditional generative AI?
Traditional generative AI generates responses solely based on training data, which can lead to inaccuracies or hallucinations. Conversely, RAG retrieves real-time or pre-validated data from external knowledge bases before generating a response. This grounding step ensures that RAG outputs are contextually accurate and up-to-date, making it more reliable for applications where factual accuracy is critical.